mirror of
https://github.com/YGGverse/xash3d-fwgs.git
synced 2025-01-22 12:54:13 +00:00
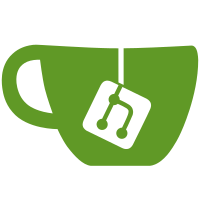
The `.editorconfig` file in this repo is configured to trim all trailing whitespace regardless of whether the line is modified. Trims all trailing whitespace in the repository to make the codebase easier to work with in editors that respect `.editorconfig`. `git blame` becomes less useful on these lines but it already isn't very useful. Commands: ``` find . -type f -name '*.h' -exec sed --in-place 's/[[:space:]]\+$//' {} \+ find . -type f -name '*.c' -exec sed --in-place 's/[[:space:]]\+$//' {} \+ ```
472 lines
10 KiB
C
472 lines
10 KiB
C
/*
|
|
img_quant.c - image quantizer. based on Antony Dekker original code
|
|
Copyright (C) 2011 Uncle Mike
|
|
|
|
This program is free software: you can redistribute it and/or modify
|
|
it under the terms of the GNU General Public License as published by
|
|
the Free Software Foundation, either version 3 of the License, or
|
|
(at your option) any later version.
|
|
|
|
This program is distributed in the hope that it will be useful,
|
|
but WITHOUT ANY WARRANTY; without even the implied warranty of
|
|
MERCHANTABILITY or FITNESS FOR A PARTICULAR PURPOSE. See the
|
|
GNU General Public License for more details.
|
|
*/
|
|
|
|
#include "imagelib.h"
|
|
|
|
|
|
#define netsize 256 // number of colours used
|
|
#define prime1 499
|
|
#define prime2 491
|
|
#define prime3 487
|
|
#define prime4 503
|
|
|
|
#define minpicturebytes (3*prime4) // minimum size for input image
|
|
|
|
#define maxnetpos (netsize-1)
|
|
#define netbiasshift 4 // bias for colour values
|
|
#define ncycles 100 // no. of learning cycles
|
|
|
|
// defs for freq and bias
|
|
#define intbiasshift 16 // bias for fractions
|
|
#define intbias (1<<intbiasshift)
|
|
#define gammashift 10 // gamma = 1024
|
|
#define gamma (1<<gammashift)
|
|
#define betashift 10
|
|
#define beta (intbias>>betashift) // beta = 1 / 1024
|
|
#define betagamma (intbias<<(gammashift - betashift))
|
|
|
|
// defs for decreasing radius factor
|
|
#define initrad (netsize>>3) // for 256 cols, radius starts
|
|
#define radiusbiasshift 6 // at 32.0 biased by 6 bits
|
|
#define radiusbias (1<<radiusbiasshift)
|
|
#define initradius (initrad * radiusbias) // and decreases by a
|
|
#define radiusdec 30 // factor of 1/30 each cycle
|
|
|
|
// defs for decreasing alpha factor
|
|
#define alphabiasshift 10 // alpha starts at 1.0
|
|
#define initalpha (1<<alphabiasshift)
|
|
int alphadec; // biased by 10 bits
|
|
|
|
// radbias and alpharadbias used for radpower calculation
|
|
#define radbiasshift 8
|
|
#define radbias (1<<radbiasshift)
|
|
#define alpharadbshift (alphabiasshift+radbiasshift)
|
|
#define alpharadbias (1<<alpharadbshift)
|
|
|
|
// types and global variables
|
|
static byte *thepicture; // the input image itself
|
|
static int lengthcount; // lengthcount = H*W*3
|
|
static int samplefac; // sampling factor 1..30
|
|
static int network[netsize][4]; // the network itself
|
|
static int netindex[256]; // for network lookup - really 256
|
|
static int bias[netsize]; // bias and freq arrays for learning
|
|
static int freq[netsize];
|
|
static int radpower[initrad]; // radpower for precomputation
|
|
|
|
void initnet( byte *thepic, int len, int sample )
|
|
{
|
|
register int i, *p;
|
|
|
|
thepicture = thepic;
|
|
lengthcount = len;
|
|
samplefac = sample;
|
|
|
|
for( i = 0; i < netsize; i++ )
|
|
{
|
|
p = network[i];
|
|
p[0] = p[1] = p[2] = (i << (netbiasshift + 8)) / netsize;
|
|
freq[i] = intbias / netsize; // 1 / netsize
|
|
bias[i] = 0;
|
|
}
|
|
}
|
|
|
|
// Unbias network to give byte values 0..255 and record position i to prepare for sort
|
|
void unbiasnet( void )
|
|
{
|
|
int i, j, temp;
|
|
|
|
for( i = 0; i < netsize; i++ )
|
|
{
|
|
for( j = 0; j < 3; j++ )
|
|
{
|
|
// OLD CODE: network[i][j] >>= netbiasshift;
|
|
// Fix based on bug report by Juergen Weigert jw@suse.de
|
|
temp = (network[i][j] + (1 << (netbiasshift - 1))) >> netbiasshift;
|
|
if( temp > 255 ) temp = 255;
|
|
network[i][j] = temp;
|
|
}
|
|
|
|
network[i][3] = i; // record colour num
|
|
}
|
|
}
|
|
|
|
// Insertion sort of network and building of netindex[0..255] (to do after unbias)
|
|
void inxbuild( void )
|
|
{
|
|
register int *p, *q;
|
|
register int i, j, smallpos, smallval;
|
|
int previouscol, startpos;
|
|
|
|
previouscol = 0;
|
|
startpos = 0;
|
|
|
|
for( i = 0; i < netsize; i++ )
|
|
{
|
|
p = network[i];
|
|
smallpos = i;
|
|
smallval = p[1]; // index on g
|
|
|
|
// find smallest in i..netsize-1
|
|
for( j = i + 1; j < netsize; j++ )
|
|
{
|
|
q = network[j];
|
|
if( q[1] < smallval )
|
|
{
|
|
// index on g
|
|
smallpos = j;
|
|
smallval = q[1]; // index on g
|
|
}
|
|
}
|
|
|
|
q = network[smallpos];
|
|
|
|
// swap p (i) and q (smallpos) entries
|
|
if( i != smallpos )
|
|
{
|
|
j = q[0]; q[0] = p[0]; p[0] = j;
|
|
j = q[1]; q[1] = p[1]; p[1] = j;
|
|
j = q[2]; q[2] = p[2]; p[2] = j;
|
|
j = q[3]; q[3] = p[3]; p[3] = j;
|
|
}
|
|
|
|
// smallval entry is now in position i
|
|
if( smallval != previouscol )
|
|
{
|
|
netindex[previouscol] = (startpos+i) >> 1;
|
|
|
|
for( j = previouscol + 1; j < smallval; j++ )
|
|
netindex[j] = i;
|
|
|
|
previouscol = smallval;
|
|
startpos = i;
|
|
}
|
|
}
|
|
|
|
netindex[previouscol] = (startpos + maxnetpos)>>1;
|
|
|
|
for( j = previouscol + 1; j < 256; j++ )
|
|
netindex[j] = maxnetpos; // really 256
|
|
}
|
|
|
|
|
|
// Search for BGR values 0..255 (after net is unbiased) and return colour index
|
|
int inxsearch( int r, int g, int b )
|
|
{
|
|
register int i, j, dist, a, bestd;
|
|
register int *p;
|
|
int best;
|
|
|
|
bestd = 1000; // biggest possible dist is 256 * 3
|
|
best = -1;
|
|
i = netindex[g]; // index on g
|
|
j = i - 1; // start at netindex[g] and work outwards
|
|
|
|
while(( i < netsize ) || ( j >= 0 ))
|
|
{
|
|
if( i < netsize )
|
|
{
|
|
p = network[i];
|
|
dist = p[1] - g; // inx key
|
|
|
|
if( dist >= bestd )
|
|
{
|
|
i = netsize; // stop iter
|
|
}
|
|
else
|
|
{
|
|
i++;
|
|
if( dist < 0 ) dist = -dist;
|
|
a = p[2] - b;
|
|
if( a < 0 ) a = -a;
|
|
dist += a;
|
|
|
|
if( dist < bestd )
|
|
{
|
|
a = p[0] - r;
|
|
if( a < 0 ) a = -a;
|
|
dist += a;
|
|
|
|
if( dist < bestd )
|
|
{
|
|
bestd = dist;
|
|
best = p[3];
|
|
}
|
|
}
|
|
}
|
|
}
|
|
|
|
if( j >= 0 )
|
|
{
|
|
p = network[j];
|
|
dist = g - p[1]; // inx key - reverse dif
|
|
|
|
if( dist >= bestd )
|
|
{
|
|
j = -1; // stop iter
|
|
}
|
|
else
|
|
{
|
|
j--;
|
|
if( dist < 0 ) dist = -dist;
|
|
a = p[2] - b;
|
|
if( a < 0 ) a = -a;
|
|
dist += a;
|
|
|
|
if( dist < bestd )
|
|
{
|
|
a = p[0] - r;
|
|
if( a < 0 ) a = -a;
|
|
dist += a;
|
|
if( dist < bestd )
|
|
{
|
|
bestd = dist;
|
|
best = p[3];
|
|
}
|
|
}
|
|
}
|
|
}
|
|
}
|
|
|
|
return best;
|
|
}
|
|
|
|
// Search for biased BGR values
|
|
int contest( int r, int g, int b )
|
|
{
|
|
register int *p, *f, *n;
|
|
register int i, dist, a, biasdist, betafreq;
|
|
int bestpos, bestbiaspos, bestd, bestbiasd;
|
|
|
|
// finds closest neuron (min dist) and updates freq
|
|
// finds best neuron (min dist-bias) and returns position
|
|
// for frequently chosen neurons, freq[i] is high and bias[i] is negative
|
|
// bias[i] = gamma * ((1 / netsize) - freq[i])
|
|
bestd = INT_MAX;
|
|
bestbiasd = bestd;
|
|
bestpos = -1;
|
|
bestbiaspos = bestpos;
|
|
p = bias;
|
|
f = freq;
|
|
|
|
for( i = 0; i < netsize; i++ )
|
|
{
|
|
n = network[i];
|
|
dist = n[2] - b;
|
|
if( dist < 0 ) dist = -dist;
|
|
a = n[1] - g;
|
|
if( a < 0 ) a = -a;
|
|
dist += a;
|
|
a = n[0] - r;
|
|
if( a < 0 ) a = -a;
|
|
dist += a;
|
|
|
|
if( dist < bestd )
|
|
{
|
|
bestd = dist;
|
|
bestpos = i;
|
|
}
|
|
|
|
biasdist = dist - ((*p) >> (intbiasshift - netbiasshift));
|
|
|
|
if( biasdist < bestbiasd )
|
|
{
|
|
bestbiasd = biasdist;
|
|
bestbiaspos = i;
|
|
}
|
|
|
|
betafreq = (*f >> betashift);
|
|
*f++ -= betafreq;
|
|
*p++ += (betafreq << gammashift);
|
|
}
|
|
|
|
freq[bestpos] += beta;
|
|
bias[bestpos] -= betagamma;
|
|
|
|
return bestbiaspos;
|
|
}
|
|
|
|
// Move neuron i towards biased (b,g,r) by factor alpha
|
|
void altersingle( int alpha, int i, int r, int g, int b )
|
|
{
|
|
register int *n;
|
|
|
|
n = network[i]; // alter hit neuron
|
|
*n -= (alpha * (*n - r)) / initalpha;
|
|
n++;
|
|
*n -= (alpha * (*n - g)) / initalpha;
|
|
n++;
|
|
*n -= (alpha * (*n - b)) / initalpha;
|
|
}
|
|
|
|
// Move adjacent neurons by precomputed alpha*(1-((i-j)^2/[r]^2)) in radpower[|i-j|]
|
|
void alterneigh( int rad, int i, int r, int g, int b )
|
|
{
|
|
register int j, k, lo, hi, a;
|
|
register int *p, *q;
|
|
|
|
lo = i - rad;
|
|
if( lo < -1 ) lo = -1;
|
|
hi = i + rad;
|
|
if( hi > netsize ) hi = netsize;
|
|
|
|
j = i + 1;
|
|
k = i - 1;
|
|
q = radpower;
|
|
|
|
while(( j < hi ) || ( k > lo ))
|
|
{
|
|
a = (*(++q));
|
|
|
|
if( j < hi )
|
|
{
|
|
p = network[j];
|
|
*p -= (a * (*p - r)) / alpharadbias;
|
|
p++;
|
|
*p -= (a * (*p - g)) / alpharadbias;
|
|
p++;
|
|
*p -= (a * (*p - b)) / alpharadbias;
|
|
j++;
|
|
}
|
|
|
|
if( k > lo )
|
|
{
|
|
p = network[k];
|
|
*p -= (a * (*p - r)) / alpharadbias;
|
|
p++;
|
|
*p -= (a * (*p - g)) / alpharadbias;
|
|
p++;
|
|
*p -= (a * (*p - b)) / alpharadbias;
|
|
k--;
|
|
}
|
|
}
|
|
}
|
|
|
|
// Main Learning Loop
|
|
void learn( void )
|
|
{
|
|
register byte *p;
|
|
register int i, j, r, g, b;
|
|
int radius, rad, alpha, step;
|
|
int delta, samplepixels;
|
|
byte *lim;
|
|
|
|
alphadec = 30 + ((samplefac - 1) / 3);
|
|
p = thepicture;
|
|
lim = thepicture + lengthcount;
|
|
samplepixels = lengthcount / (image.bpp * samplefac);
|
|
delta = samplepixels / ncycles;
|
|
alpha = initalpha;
|
|
radius = initradius;
|
|
|
|
rad = radius >> radiusbiasshift;
|
|
if( rad <= 1 ) rad = 0;
|
|
|
|
for( i = 0; i < rad; i++ )
|
|
radpower[i] = alpha * ((( rad * rad - i * i ) * radbias ) / ( rad * rad ));
|
|
|
|
if( delta <= 0 ) return;
|
|
|
|
if(( lengthcount % prime1 ) != 0 )
|
|
{
|
|
step = prime1 * image.bpp;
|
|
}
|
|
else if(( lengthcount % prime2 ) != 0 )
|
|
{
|
|
step = prime2 * image.bpp;
|
|
}
|
|
else if(( lengthcount % prime3 ) != 0 )
|
|
{
|
|
step = prime3 * image.bpp;
|
|
}
|
|
else
|
|
{
|
|
step = prime4 * image.bpp;
|
|
}
|
|
|
|
i = 0;
|
|
|
|
while( i < samplepixels )
|
|
{
|
|
r = p[0] << netbiasshift;
|
|
g = p[1] << netbiasshift;
|
|
b = p[2] << netbiasshift;
|
|
j = contest( r, g, b );
|
|
|
|
altersingle( alpha, j, r, g, b );
|
|
if( rad ) alterneigh( rad, j, r, g, b ); // alter neighbours
|
|
|
|
p += step;
|
|
if( p >= lim ) p -= lengthcount;
|
|
|
|
i++;
|
|
|
|
if( i % delta == 0 )
|
|
{
|
|
alpha -= alpha / alphadec;
|
|
radius -= radius / radiusdec;
|
|
rad = radius >> radiusbiasshift;
|
|
if( rad <= 1 ) rad = 0;
|
|
|
|
for( j = 0; j < rad; j++ )
|
|
radpower[j] = alpha * ((( rad * rad - j * j ) * radbias ) / ( rad * rad ));
|
|
}
|
|
}
|
|
}
|
|
|
|
// returns the actual number of palette entries.
|
|
rgbdata_t *Image_Quantize( rgbdata_t *pic )
|
|
{
|
|
int i;
|
|
|
|
// quick case to reject unneeded conversions
|
|
if( pic->type == PF_INDEXED_24 || pic->type == PF_INDEXED_32 )
|
|
return pic;
|
|
|
|
Image_CopyParms( pic );
|
|
image.size = image.width * image.height;
|
|
image.bpp = PFDesc[pic->type].bpp;
|
|
image.ptr = 0;
|
|
|
|
// allocate 8-bit buffer
|
|
image.tempbuffer = Mem_Realloc( host.imagepool, image.tempbuffer, image.size );
|
|
|
|
initnet( pic->buffer, pic->size, 10 );
|
|
learn();
|
|
unbiasnet();
|
|
|
|
pic->palette = Mem_Malloc( host.imagepool, netsize * 3 );
|
|
|
|
for( i = 0; i < netsize; i++ )
|
|
{
|
|
pic->palette[i*3+0] = network[i][0]; // red
|
|
pic->palette[i*3+1] = network[i][1]; // green
|
|
pic->palette[i*3+2] = network[i][2]; // blue
|
|
}
|
|
|
|
inxbuild();
|
|
|
|
for( i = 0; i < image.width * image.height; i++ )
|
|
{
|
|
image.tempbuffer[i] = inxsearch( pic->buffer[i*image.bpp+0], pic->buffer[i*image.bpp+1], pic->buffer[i*image.bpp+2] );
|
|
}
|
|
|
|
pic->buffer = Mem_Realloc( host.imagepool, pic->buffer, image.size );
|
|
memcpy( pic->buffer, image.tempbuffer, image.size );
|
|
pic->type = PF_INDEXED_24;
|
|
pic->size = image.size;
|
|
|
|
return pic;
|
|
}
|